Preventing Fraud in Finance
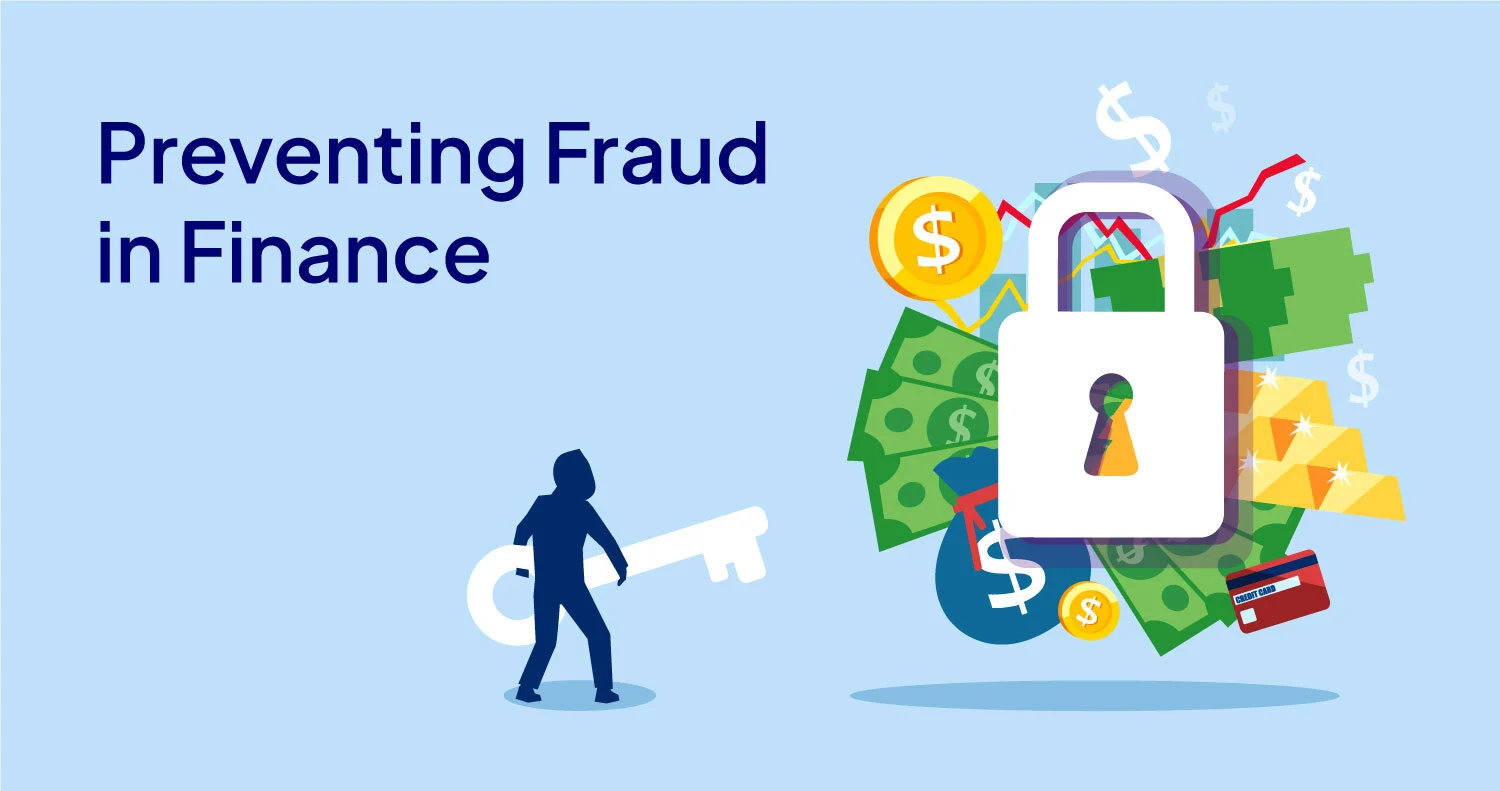
Get Easy & Instant Credit Now
Nurture your financial health with Potlee
With advancing technology and the digitization of financial systems, the landscape of fraud in finance is shifting. Cyber threats and cybersecurity risks are increasing as digital channels for financial transactions become more prevalent. Fraudsters are leveraging AI and automation to execute sophisticated fraud schemes, using bots to bypass security measures, create fake accounts and conduct large-scale fraudulent transactions. Social engineering and phishing attacks remain prevalent, with fraudsters using tactics such as impersonation, deception and manipulation to trick individuals into revealing sensitive information or transferring funds to fraudulent accounts. Here are some numbers in the context of India to give you an idea about the scale of financial fraud.
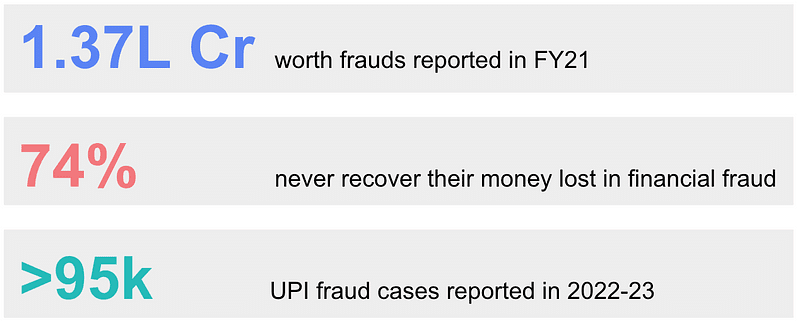
Down the memory lane of recent frauds
- In the 2018 "Nirav Modi-PNB scam," around $2 billion was fraudulently obtained using fake letters of credit (LoCs) issued by Punjab National Bank (PNB), a major public sector bank in India. The system failed to flag Nirav Modi as a defaulter when he initially defaulted on a payment of Rs. 800 crore
- The famous web series “Jamtara - Sabka Number Ayega” is based on the true events of a phishing scam that took place in the town of Jamtara in Jharkhand, India
- In the 2017 Equifax data breach, the personal information of 143 million individuals was compromised, resulting in significant identity theft and fraud. The estimated costs for Equifax reached up to $600 million
- In 2019, India's Aadhaar biometric identification system faced multiple instances of fraud where fake Aadhaar cards were used for fraudulent transactions. The government had to implement additional security measures and invest in AI-based fraud detection technologies to enhance KYC processes and prevent further fraud in the system that contains the personal information of over 1.2 billion individuals
- Transaction fraud: In 2020, the CEO of a UK-based energy firm fell victim to a Business Email Compromise (BEC) scam, resulting in a loss of approximately $243,000. The fraud involved the fraudulent interception of email communication related to a pending transaction and the funds were transferred to a fraudulent bank account based on the false information provided by the scammers
Common types of financial fraud
Various factors, including insider collusion, identity theft, money laundering and cyberattacks can cause fraud in the financial industry. Financial fraud can manifest in multiple forms, from traditional schemes to sophisticated, technology-driven methods. Some common types of financial fraud include:
Types of cyber financial fraudDescriptionPhishingSending fake emails, messages, or websites to trick individuals into revealing personal/financial information; 2019 SBI Phishing ScamCard skimmingInstalling devices on card readers/ATMs to capture card information for fraudulent use; Axis Bank ATM Skimming CaseBusiness email compromise (BEC)Impersonating a legitimate business to deceive employees into transferring funds to fake accounts; BEC Scam at Pune-based Cosmos BankSocial engineeringManipulating individuals through psychological techniques to gain access to financial information/funds
Existing fraud models are not enough to counter fraud
LexisNexis Risk Solutions has determined that existing fraud detection models are insufficient in detecting a significant portion of synthetic identities, ranging from 85% to 95%. This is due to limitations such as a lack of real-time insights, inadequate support for comprehensive telemetry data spanning years of transaction activity and resulting inaccurate model results.
Currently fraud prevention are reactive in nature rather than proactive. CISOs have expressed the need for more advanced and intuitive fraud prevention modeling applications and tools to counter identity fraud effectively as organizations increasingly onboard more fraud prevention analysts in response to the escalating risks.
How can AI detect and prevent fraud?
AI is increasingly being utilized by fraudsters to carry out sophisticated and advanced fraud schemes. With the advancement of technology, fraudsters are leveraging AI and automation to bypass security measures, create fake accounts and conduct fraudulent transactions on a large scale. This has resulted in a growing need for more advanced cyber solutions to detect and prevent fraud.
To combat the rising threat of advanced fraud, AI is also being used by financial institutions and companies for fraud detection and prevention. According to a survey by The Economist, 58% of big banks heavily use AI in fraud detection, while 32% use it to some extent. The demand for AI-based security products is also on the rise, with the market estimated to reach $133.8 billion by 2030, as per Acumen Research and Consulting report.
While AI may not provide 100% accuracy or unbiased decisions, it can help build fraud detection systems with a certain level of accuracy. Indian banks such as SBI, HDFC, ICICI, etc. use generative AI algorithms to analyze real-time transaction data and detect potentially fraudulent activities.
Synthetic identity fraud
Synthetic identity fraud is a type of identity fraud where criminals create new identities by combining real and fake information to establish fraudulent accounts or obtain credit or other financial benefits. Unlike traditional identity fraud, where a fraudster steals and uses someone else's personal information, synthetic identity fraud involves creating entirely new identities that do not belong to any actual person.
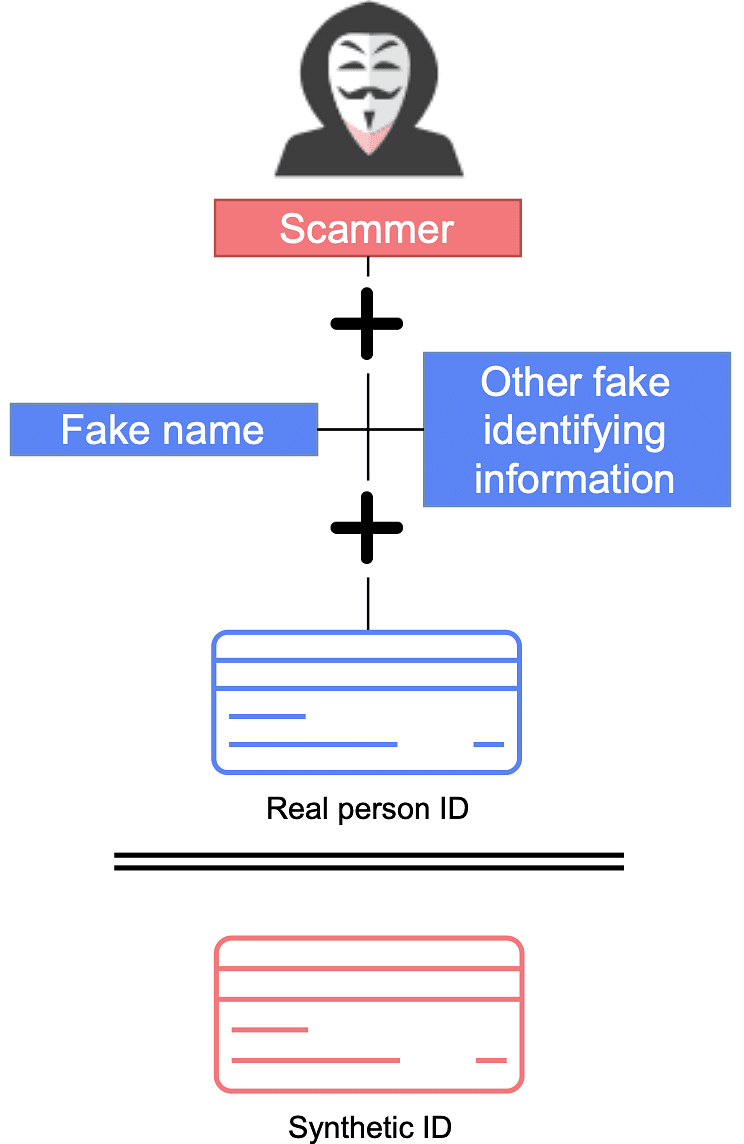
Using AI to prevent synthetic identity fraud
Leading AI providers like Experian, Ikata, Kount, LexisNexis Risk Solutions and Telesign use decades of data to train their models and assign trust scores for identity fraud prevention. For example, Telesign uses over 2,200 digital attributes and historical data patterns to create risk assessment scores in milliseconds, verifying new accounts.
AI-generated trust scores are critical in balancing customer trust and user experience (UX), helping fraud prevention analysts create effective constraint-based rules and workflows that save time and reduce false positives. This is crucial in synthetic fraud, where traditional prevention techniques are evaded. Vendors like Kount and Telesign provide trust scores based on multi-year analysis of transactions and real-time identity management, enabling more accurate fraud detection.
AI also provides contextual intelligence by integrating identity proofing, fraud detection and user authentication into a unified tech stack with AI-powered insights. This holistic approach allows for more accurate detection and prevention of identity fraud.
AI's predictive analytics and machine learning are ideal for finding anomalies in real-time identity-based activity. With more data, machine learning models have higher accuracy in fraud scoring. Advanced fraud prevention platforms can build convolutional neural networks in real-time, providing risk scoring for each transaction and thwarting identity fraud.
KYC fraud
KYC fraud refers to fraudulent activities in the financial system due to the lack of proper identification and verification of customers. This can include false identities, online schemes, money laundering and other financial crimes. A study by Thomson Reuters found that the average cost of a KYC regulatory penalty globally in 2020 was $5.2 million, with the highest penalty recorded at $5.1 billion.
Recently, with digital lending apps popping up like mushrooms, KYC frauds have become a major concern for fintech companies. People produce fake KYC documents to secure the loan.
How to prevent KYC fraud using AI?
AI (Artificial Intelligence) can utilize phone number velocity, traffic patterns and fraud database consortiums to detect and prevent KYC (Know Your Customer) fraud in the following ways:
- AI-powered algorithms can analyze the frequency and rate at which a phone number is used for various activities, such as creating multiple accounts or making repeated transactions within a short period. If the velocity exceeds normal thresholds, it can trigger alerts for further investigation, as it may indicate potential KYC fraud
- To detect abnormal or suspicious behavior, AI algorithms can analyze traffic patterns across channels, such as IP addresses, device information, or geolocation. For instance, sudden spikes in transaction volume from a specific IP address or location could indicate potential KYC fraud, where fraudsters may be manipulating geolocation or using a botnet to create fake accounts
- AI can use fraud database consortiums and collaborative efforts among organizations to share fraud-related information to cross-check customer data against known fraudsters and suspicious patterns. This enables real-time detection and prevention of KYC fraud
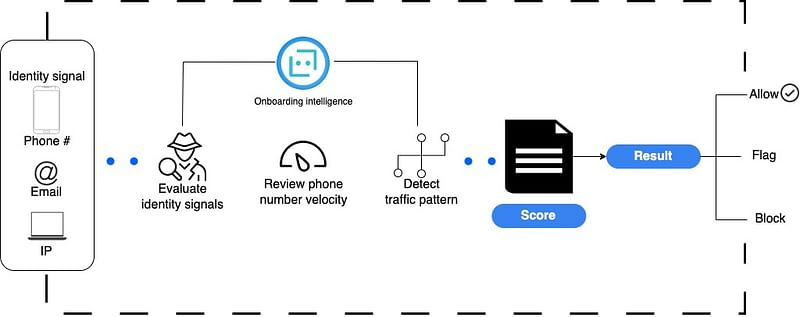
Transaction monitoring in the wild
Transaction fraud in finance refers to fraudulent activities during financial transactions, such as payments, fund transfers, or other financial exchanges. It can involve various tactics, including unauthorized access, fake invoices, fake accounts, insider fraud and other fraudulent schemes.
Companies have been marred with transaction fraud. Societe Generale: In 2008, Societe Generale, a French multinational bank, faced a massive transaction fraud scandal when it was revealed that a rogue trader had engaged in unauthorized trading and concealed the losses, resulting in a loss of approximately €4.9 billion (around $6.7 billion) for the bank.
In 2018, PNB, one of India's largest public sector banks, faced a major transaction fraud scandal involving the fraudulent issuance of Letters of Undertaking (LoUs) by certain bank officials in connivance with a group of individuals. The fraud resulted in a loss of over ₹14,000 crore (approximately $2 billion) for the bank.
Fraud transactions are not only limited to financial institutions only. They are also very prevalent in eCommerce. Online scams involving Unified Payments Interface (UPI) and QR codes have become increasingly common on e-commerce platforms like OLX, where fraudsters exploit users' lack of knowledge about online payments.
Transaction Alert!
Some examples of transaction monitoring alerts include:
- Irregular changes in customer balance or account activity
- Unusual transfers between accounts owned by the same person
- Suspicious trades based on correlations between previously unrelated assets
- Unusually large sums are moved out of an account without prior notice
- Unexpected bank transfers from foreign countries
Preventing transaction fraud using AI
- Anomaly detection: AI-based anomaly detection analyzes data patterns to detect fraud. For UPI and QR code scams, AI can identify unusual patterns like multiple transactions to the same QR code from different users or transactions that deviate from normal behavior, triggering alerts for investigation
- Behavioral analytics: AI analyzes user behavior data to establish baseline behavior and detect deviations that may indicate fraud. For example, sudden multiple transactions to unfamiliar QR codes or receiving payments from various sources in a short period may trigger alerts for investigation
- Real-time monitoring: AI can enable real-time monitoring of transactions and user interactions, allowing quick detection and response to potential fraud attempts. AI-powered systems can continuously analyze data streams, such as transaction data, user behavior data and communication data, in real time and raise alerts or block suspicious transactions before they are completed
Paytm utilizes Pi, an AI and ML-powered fraud and risk management platform, to categorize customers into risk tiers in lending scenarios. It analyzes IP addresses, transaction details, locations, times and historical transactions to identify unusual patterns and outliers, protecting customer accounts from fraud.
Razorpay, a leading player in the digital payments industry, employs Thirdwatch, an advanced AI and machine learning solution, to help merchants detect risky users, fraudulent orders and impulse purchases.
Balancing Act
The use of AI in fraud detection is a balancing act. AI can analyze data, detect anomalies and flag potential fraud, protecting businesses and customers. This helps companies to protect themselves and their customers from financial losses and reputational damage. However, it can also generate friction for authentic users, impacting user experience and satisfaction. Striking the right balance is crucial to prevent fraud without hindering legitimate transactions or causing customer inconvenience.
This requires careful consideration of AI-powered systems, using robust models and algorithms, regular monitoring and refining, and ongoing assessment of their impact on user experience and business objectives. Ultimately, the goal is to leverage AI to combat fraud effectively while minimizing any adverse effect on genuine users, thus achieving a harmonious balance between fraud prevention and user experience in the digital landscape.
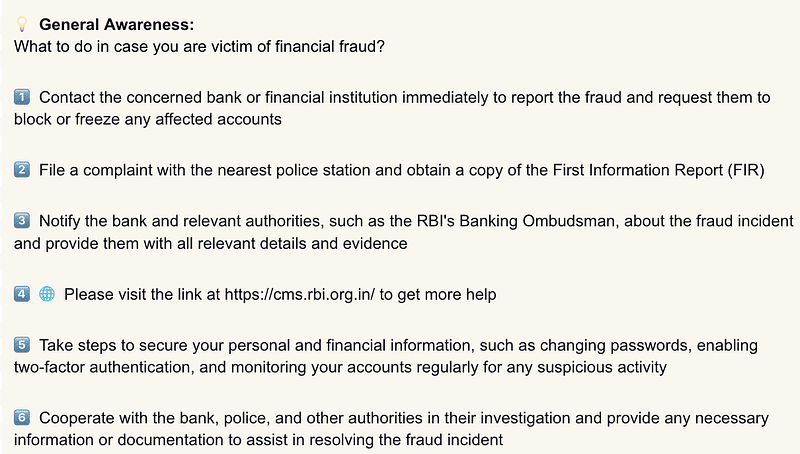
Get Easy & Instant Credit Now
Nurture your financial health with Potlee